This course is designed to help you master dbt (Data Build Tool) through hands-on practice. Rather than just theory, we’ll walk through real-world examples, applying dbt’s features step by step.
You’ll start with the fundamentals—understanding dbt’s architecture—before progressing to advanced transformations, testing, performance optimization, and automation. Throughout the course, we will build a fully functional analytics pipeline for an e-commerce business, applying dbt features at each stage.
Who Is This Course For? #
- Data Analysts
- Data Engineers
- Business Intelligence Professionals
- Anyone working with SQL-based transformations
Prerequisites #
- Basic knowledge of SQL
- Familiarity with Data Warehousing concepts (optional)
- Understanding of ETL/ELT workflows (optional)
Course Curriculum #
Module 1: Introduction to dbt #
- What is dbt and why is it important?
- dbt vs. traditional ETL tools
- The dbt workflow (ELT paradigm)
- dbt Core vs. dbt Cloud
Module 2: Setting Up Your dbt Environment #
- Installing dbt (Local vs. Cloud)
- Setting up dbt with:
- Postgres
- Snowflake
- BigQuery
- Redshift
- Configuring the
profiles.yml
file
Module 3: Working with dbt Models #
- Understanding models in dbt
- Writing SQL transformations in dbt
- Materializations: view, table, ephemeral, incremental
- Running and testing dbt models (
dbt run
,dbt test
)
Module 4: dbt Testing & Data Quality #
- The importance of data testing
- Writing dbt tests for:
- Unique constraints
- Null checks
- Referential integrity
- Custom tests using
schema.yml
- Advanced testing with Great Expectations and dbt
Module 5: dbt Documentation & Version Control #
- Generating and viewing dbt documentation (
dbt docs generate
) - Adding descriptions to models, columns, and sources
- Version control with Git + dbt
- Collaborative workflows in dbt Cloud
Module 6: Advanced dbt Concepts #
- Using seeds and sources in dbt
- Refactoring large dbt projects with macros
- Implementing hooks & operations
- Using Jinja templating in dbt
- dbt packages & dependencies
Module 7: dbt Performance Optimization #
- Understanding incremental models in dbt
- Performance tuning for:
- Snowflake
- BigQuery
- Redshift
- Postgres
- Managing dependencies in large dbt projects
- Optimizing dbt compilation & execution
Module 8: Deploying dbt in Production #
- CI/CD with dbt Cloud
- Automating dbt workflows using Airflow
- dbt integration with orchestration tools (Prefect, Dagster)
- Error handling and logging in dbt
Module 9: dbt for Analytics & BI Teams #
- How dbt fits into the Modern Data Stack
- Using dbt for data marts and business intelligence
- Integrating dbt with:
- Looker
- Metabase
- Tableau
- Best practices for maintaining dbt projects in a team setting
Module 10: Capstone Project #
- Project Goal: Build an end-to-end dbt transformation pipeline
- Tools: Choose between Postgres, Snowflake, or BigQuery
- Tasks:
- Create source tables and define staging models
- Implement incremental models
- Write tests and document your models
- Optimize for performance
- Deploy the project using dbt Cloud + CI/CD
Learning Path #
This schema outlines how you progress through the course.
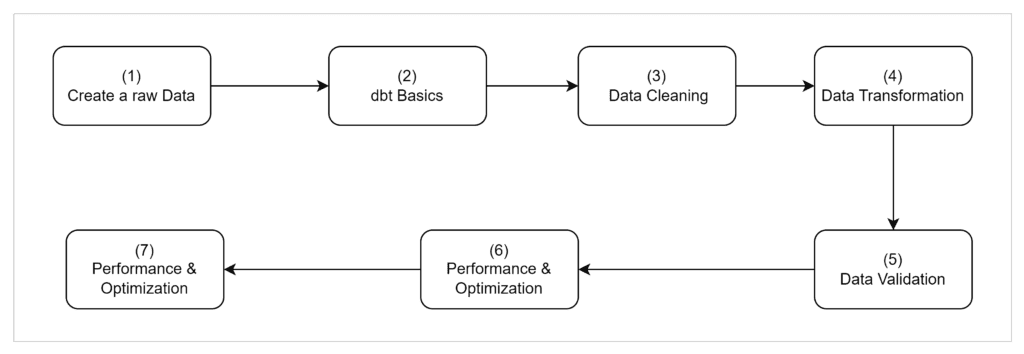
Project workflow #
This diagram illustrates how raw data will flow through the dbt models.
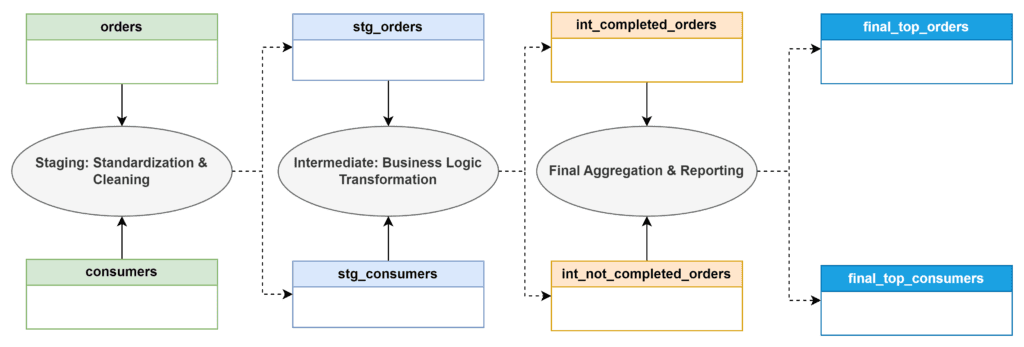
dbt Project Structure #
This schema represents how we will organize the dbt models throughout the course.
dbt_project/ │── models/ │ │── staging/ │ │ │── stg_orders.sql # Cleaned order data │ │ │── stg_consumers.sql # Cleaned consumer data │ │ │ │── intermediate/ │ │ │── int_completed_orders.sql # Filtered completed orders │ │ │── int_not_completed_orders.sql # Filtered pending orders │ │ │ │── final/ │ │ │── final_top_consumers.sql # Aggregated customer spending │ │ │── final_sales_performance.sql # Monthly revenue trends │ │── seeds/ │ │── product_categories.csv # Static reference data for products │ │── country_codes.csv # Country mapping data │ │── tests/ │ │── schema.yml # Data integrity tests (unique, not null, etc.) │ │── dbt_project.yml # Project configuration │── README.md # Documentation
Conclusion #
By following this step-by-step approach, you’ll gain practical experience with dbt while building a real-world e-commerce analytics pipeline.