Apache Spark is a unified computing engine designed for parallel data processing on clusters. It is one of the most actively developed open-source tools for big data, supporting multiple programming languages like Python, Java, Scala, and R. With built-in libraries for SQL, streaming, and machine learning, Spark is highly scalable, running efficiently from a single laptop to massive server clusters, making it a versatile choice for developers and data scientists.
Spark Components #
To understand how Spark works, let’s first explore its core components:

- Spark Core: The foundation of Spark, providing essential functionalities and APIs.
- Spark SQL: Enables querying structured data using SQL commands.
- Spark Streaming: Allows real-time data stream processing.
- MLlib: A scalable library for machine learning model development.
- GraphX: Facilitates graph processing and analytics.
Architecture #
Now that we have an overview of Spark’s components, let’s dive into its architecture and understand how it operates at scale.
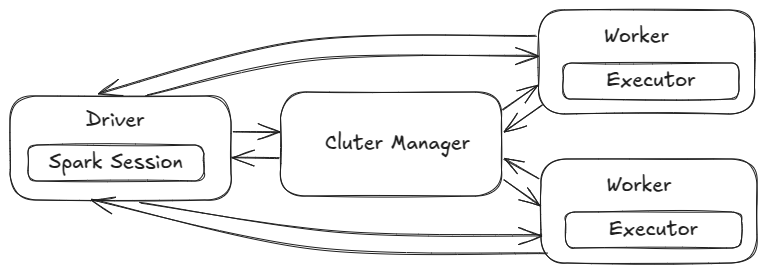
Now, let’s explore each component.
Cluster manager #
A cluster manager in Spark is a system that allocates and manages computing resources across multiple machines in a cluster. It decides how to distribute tasks and provides the necessary CPU and memory for Spark applications. Examples include Spark’s standalone cluster manager, YARN, Kubernetes and Mesos.
Spark Nodes #
Apache Spark consists of two main types of nodes that work together to execute applications efficiently.
Driver Node #
The Driver is the central component that initiates, manages, and oversees the execution of a Spark application. It:
- Creates the Spark Session.
- Distributes tasks to executors across the cluster.
- Collects and consolidates results.
- Manages communication with the cluster’s resources.
Worker Nodes #
A worker node is responsible for executing Spark jobs. It hosts executors, which are Java Virtual Machine (JVM) processes that perform the actual computations. Multiple executors can run on a single worker node, enhancing parallelism and efficiency.
Execution Modes #
Spark can run applications using different execution environments, either with its own scheduler or an external cluster manager for resource management.
- Standalone Scheduler: Spark can run using its built-in standalone scheduler, which manages a dedicated Spark cluster without requiring an external resource manager.
- Cluster Manager: Spark can also run on an external cluster manager (such as YARN, Mesos, or Kubernetes) that handles resource allocation and workload management across the cluster.
Run mode #
When running a Spark application, there are two execution modes available:
- Client Mode: The driver runs on the machine that submits the application, which can be a local machine or the master node of the cluster.
- Cluster Mode: The driver runs within the cluster, typically on one of the worker nodes, allowing for better resource management and scalability.
How Data is Handled? #
Now that we understand execution, let’s explore how Spark organizes and processes data efficiently.
Data Structures in Spark #
- Resilient Distributed Datasets (RDDs)
RDDs are the fundamental building blocks of Spark. They are fault-tolerant, parallel data structures that enable efficient distributed data processing across clusters.
- DataFrame
A DataFrame is a distributed, tabular collection of data organized into named columns. It provides a high-level abstraction for structured data operations, supporting SQL-like syntax for queries.
- DataSet
Datasets extend the DataFrame API by adding type safety and an object-oriented programming interface. They are available only in Scala and Java and help optimize performance through compile-time type checking.
Partitions #
Partitioning in Spark refers to the division of data into smaller, more manageable chunks known as partitions. Partitions are the basic units of parallelism in Spark, and they allow the framework to process different portions of the data simultaneously on different nodes in a cluster. A partition is a collection of rows that sit on one physical machine in your cluster
In the example below, the DataFrame on the left is divided into three partitions: P1, P2, and P3, with each partition containing two rows. Partition P1 is assigned to Executor 1, while P2 and P3 are assigned to Executor 2.

⚠️ SET THE RIGHT PARTITION NUMBER
Too few partitions lead to large partition sizes, potentially causing disk spills and slowing down queries. Too many partitions result in small partition sizes, leading to inefficient I/O patterns and an increased burden on the Spark task scheduler. Setting the right number of partitions is a key perfoamnce for your Spark Applications.
Transformations #
A transformation in Spark is an operation that defines how data should be processed but does not execute immediately. Instead of modifying data directly, transformations create a new DataFrame or RDD with the specified changes. Spark only processes the transformations when an action is triggered.
- Narrow Transformations
A narrow transformation means each input partition contributes to only one output partition. Since data remains on the same worker node, these transformations are faster and more efficient. Example: .filter()
, .map()
.
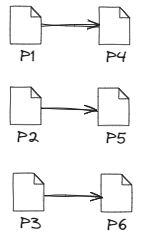
- Wide Transformations (Shuffles)
A wide transformation means data from one partition is needed by multiple output partitions, requiring data to be shuffled across the cluster. This makes them more resource-intensive. Example: .groupBy()
, .join()
.
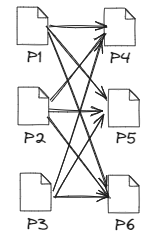
⚠️ Be aware that when using wide transformations, they can significantly slow down your applications.
Actions #
An action in Spark is a command that tells Spark to execute the computation and return a result. While transformations only define how data should be processed, actions actually trigger Spark to perform the work.
For example, calling .count()
on a DataFrame tells Spark to count the number of rows and return the result. Actions are what make Spark start processing data.
💡 The output of transformations is always an RDD, whereas the output of actions includes everything except an RDD.
Lazy evaluation #
Lazy evaluation means that Spark delays executing computations until absolutely necessary. Instead of processing data immediately when an operation is specified, Spark first builds a plan of all transformations applied to the source data. This plan is then optimized and converted into a final execution strategy just before running the job.
Why does Spark use lazy evaluation? #
This approach allows Spark to optimize the entire workflow before execution, leading to better performance. For example, Spark can apply predicate pushdown, which means it filters out unnecessary data before loading it, reducing the amount of processing needed. This makes large-scale data processing much more efficient.
Monitor and Troublshoot #
To track job progress, you can use the Spark web UI, accessible by default at the port 4040 of the driver node. This interface provides insights into Spark job status, environment details, and cluster state. Particularly valuable for tuning and debugging, the Spark UI displays essential information.

Example #
This is a simple PySpark script demonstrating how to create and manipulate a DataFrame using Spark.
from pyspark.sql import SparkSession # Create a SparkSession spark = SparkSession.builder \ .appName("SparkExample") \ .getOrCreate() # Sample data data = [("Alice", 25), ("Bob", 30), ("Charlie", 28)] columns = ["Name", "Age"] # Create a DataFrame df = spark.createDataFrame(data, columns) # Show the DataFrame df.show() # Filter data filtered_df = df.filter(df["Age"] > 25) filtered_df.show() # Stop the SparkSession spark.stop()