This is the first tutorial in the series Apache Airflow Tutorial Series: From Basics to Mastery By Examples. In this tutorial, we will cover:
- What is Apache Airflow?
- Why use Airflow?
- How does Airflow work?
- Workflow Example
- Real-world use cases
By the end of the tutorial, you’ll have a solid understanding of Airflow’s role in modern data engineering and how it helps automate workflows.
What is Apache Airflow? #
Apache Airflow is an open-source workflow orchestration tool designed for scheduling, monitoring, and managing workflows. It allows data engineers and DevOps teams to automate complex workflows, such as ETL pipelines, data processing, and machine learning workflows.
ℹ️ Think of Airflow as a task manager that automates and organizes your workflows in a systematic and scalable way.
Why Use Apache Airflow? #
Apache Airflow is widely used for its flexibility, scalability, and integration capabilities. Here’s why it’s a top choice:
- Workflow Automation
- Automates complex processes like ETL jobs, data pipelines, and machine learning tasks.
- Schedules and executes workflows on a predefined schedule (batch mode) or event triggers (when an event occurs on the external system).
- Scalability
- Can handle hundreds of tasks in parallel using different executors (Local, Celery, Kubernetes).
- Distributes workloads efficiently across multiple workers.
- Extensibility
- Provides built-in Operators and Hooks for integrations with AWS, GCP, Azure, Kubernetes, Apache Spark, etc.
- Supports custom Python scripts to define complex logic.
- Monitoring and Logging
- Offers a web-based UI to track DAG execution, logs, and task status.
- Alerts and retries help handle failures automatically.
- Open-Source and Community-Driven
- Free to use with strong community support and frequent updates.
ℹ️ In short, Airflow helps automate and manage workflows efficiently, ensuring smooth and scalable data operations.
How Does Apache Airflow Work ? #
To understand how Airflow operates, we’ll first define its main components, next explore how workflows are executed, and finally see it in action with an example.
Airflow Components #
The main components that make up Apache Airflow are as follows:
Component | Description |
---|---|
DAG (Directed Acyclic Graph) | A workflow represented as a graph of tasks. |
Operator | Defines a single task in a DAG (e.g., BashOperator, PythonOperator). |
Task | A unit of work in a DAG (executed using an Operator). |
Scheduler | Determines when and how tasks should run. |
Executor | Runs the tasks (LocalExecutor, CeleryExecutor, KubernetesExecutor). |
Web UI | Provides a user-friendly dashboard to monitor and manage DAGs. |
Metadata Database | Stores DAG execution history and task states. |
Workflow Execution Flow #
Below are the key steps involved in how Airflow processes and executes workflows:
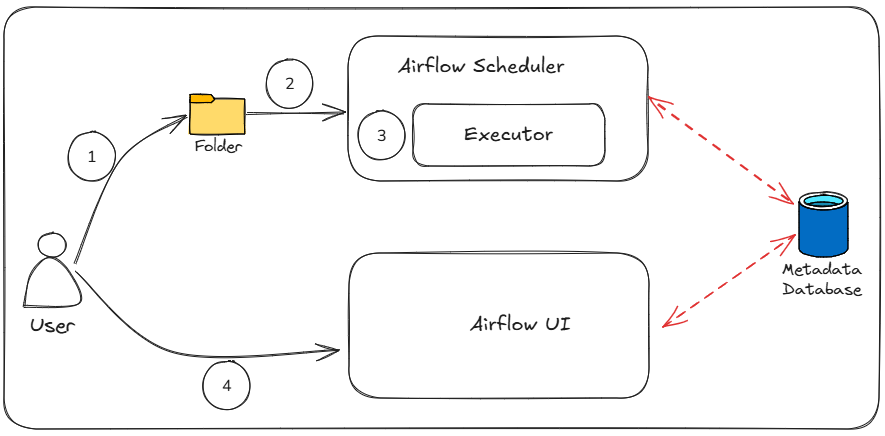
- Define the DAG: Create a workflow using Python and place it in the DAG folder.
- Schedule Execution: The Airflow Scheduler detects the DAG, schedules it, and stores metadata in the database.
- Run Tasks: Executors assign tasks to workers, running them sequentially or in parallel based on dependencies.
- Monitor & Manage: The Airflow UI provides real-time status, logs, and manual control over DAG execution.
Workflow Example #
To summarize the key points from the previous step, an ETL workflow consists of three main tasks: Extract, Transform, and Load (ETL), where each step relies on the one before it. For instance, the loading process depends on transformation, which itself requires extraction. These task dependencies form Directed Acyclic Graph (DAG), the core structure representing data pipelines in Apache Airflow.
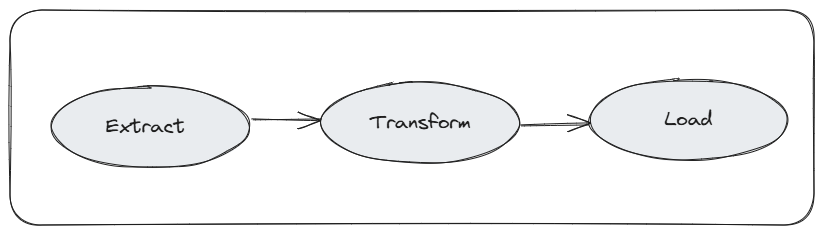
Now, let’s convert this process into a DAG (Directed Acyclic Graph) in Python.
from airflow import DAG from airflow.operators.python import PythonOperator from datetime import datetime # Define Python functions for ETL tasks def extract(): print("Extracting data...") def transform(): print("Transforming data...") def load(): print("Loading data...") # Define the DAG with DAG( dag_id='etl_example', schedule='@daily', start_date=datetime(2025, 2, 16) ) as dag: # Define tasks extract_task = PythonOperator( task_id='extract_task', python_callable=extract, # Calls the extract function dag=dag ) transform_task = PythonOperator( task_id='transform_task', python_callable=transform, # Calls the transform function dag=dag ) load_task = PythonOperator( task_id='load_task', python_callable=load, # Calls the load function dag=dag ) # Set task dependencies: Extract → Transform → Load extract_task >> transform_task >> load_task
Below is an explanation of what the above code does:
- Defining a DAG (Directed Acyclic Graph)
dag_id='etl_example'
: The unique identifier for the DAG within an Airflow instance.schedule='@daily'
: Defines the execution frequency, in this case, once per day.start_date
: Specifies when the DAG’s first execution will occur.
- Defining the tasks
- We use
PythonOperator
to execute Python functions inside Airflow. - Each task is assigned a task_id (
extract_task
,transform_task
,load_task
). - The
python_callable
parameter tells Airflow which function to run.
- We use
- Setting Dependencies
extract_task >> transform_task >> load_task
means: first, extract the data, then, transform it, and finally, load it.
Real-World Use Cases of Apache Airflow #
Below are some use cases where Apache Airflow can be effectively utilized:
- ETL & Data Pipeline Automation: Extract, transform, and load data from different sources.
- Machine Learning Pipelines: Train and deploy ML models automatically.
- Data Orchestration: Manage dependencies across Apache Spark, Hadoop, or Cloud storage.
- DevOps & CI/CD: Automate deployment workflows for data applications.
- Cloud Integration: Schedule and manage workflows on AWS, GCP, Azure.
Conclusion #
Apache Airflow is a powerful, flexible, and scalable workflow orchestration tool used across industries for automating complex workflows. By understanding what it’s used, why it’s useful, and how it works, you now have a solid foundation to start building workflows.
In the next tutorial, we’ll walk through installing Apache Airflow step by step.