In this lesson, we will explore dbt (Data Build Tool)—a powerful framework for transforming, testing, and documenting data within modern data pipelines.
We’ll cover:
- What dbt is and how it fits into the data transformation process
- Why dbt is essential for scalable, version-controlled, and efficient data modeling
- How to implement dbt in your projects, from setup to best practices
By the end of this lesson, you’ll have a clear understanding of dbt’s role in analytics engineering and how to leverage it for better, faster, and more reliable data workflows.
What is dbt? #
dbt (Data Build Tool) is a transformation workflow tool that helps teams use SQL to define, test, and document data models in a structured way.
dbt processes and executes your analytics code on your data platform. This allows you and your team to collaborate on a unified source of truth for metrics, insights, and business definitions. This consistency, along with the ability to define data tests, helps minimize errors when logic changes. It also provides alerts when issues occur.
How to Use it? #
dbt offers two approaches for running analytics workflows, each catering to different needs:
- dbt Cloud: a fully managed service, simplifies deployment, scheduling, and collaboration. As a result, teams can focus on building data models without worrying about infrastructure.
- dbt Core: an open-source command-line tool, provides full control, allowing users to run dbt models locally or within their own infrastructure. This approach is ideal for those who prefer a self-managed environment.
Regardless of the option chosen, both solutions enable teams to efficiently transform and test data, ensuring a single source of truth for metrics, insights, and business definitions.
Why Use it? #
- Eliminate redundant Data Manipulation Language (DML) and Data Definition Language (DDL) by automating transactions, table management, and schema changes. Simply define your business logic using a SQL
SELECT
statement or a Python DataFrame to generate the required dataset. dbt handles the materialization process for you. - Develop reusable, modular data models that can be referenced in future analyses. This eliminates the need to start from raw data each time.
- Optimize query performance by leveraging metadata to identify slow-running models and implementing incremental models, which dbt makes easy to configure.
- Keep your code clean and maintainable by using macros, hooks, and package management to follow the DRY (Don’t Repeat Yourself) principle.
dbt Workflow #
- Extract and load raw data into a data warehouse (ETL/ELT).
- Use dbt to transform the raw data into structured, analytics-ready tables.
- Test and document the transformed data.
- Deploy dbt models and automate execution.
This structured approach ensures efficient data transformation while maintaining accuracy and consistency.
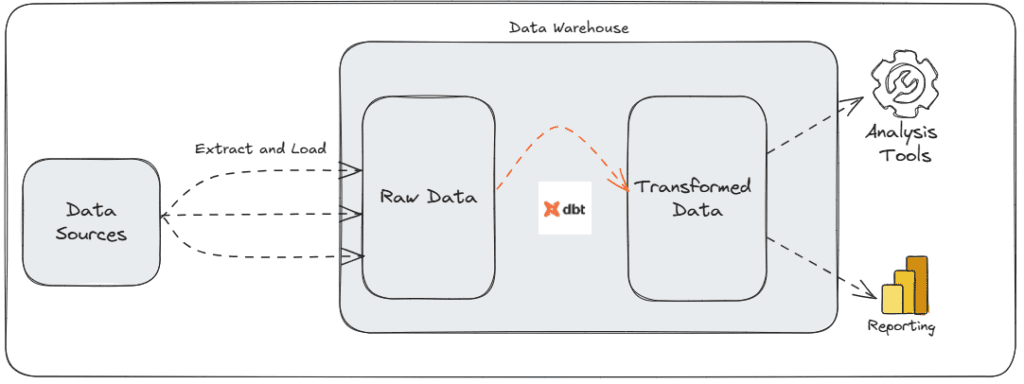
Key Features #
dbt provides a range of features to streamline data transformation, improve efficiency, and enhance collaboration. Here are some key capabilities:
- Materializing Queries Efficiently: Configure how queries are built and stored using materializations, which wrap SQL logic to create or update relations automatically.
- Code Compilation with Jinja: Use Jinja templating in SQL to introduce control structures like loops and conditionals, improving reusability through macros.
- Controlled Model Execution: Manage dependencies between transformations using the
ref
function, enabling a structured, staged approach. - Project Documentation: Auto-generate and version-control documentation, adding descriptions for models and fields in plain text or markdown.
- Model Testing: Validate SQL logic with built-in tests to ensure data integrity, executing them via the Cloud IDE or command line.
- Package Management: Use and share reusable code libraries with a built-in package manager that supports public and private repositories.
- Loading Seed Files: Store and import static or infrequently changing data (e.g., mapping country codes) as CSV files using the
seed
command. - Snapshot Data for Historical Tracking: Capture point-in-time snapshots of mutable records, preserving historical changes for analysis.