In this tutorial, we will explore the capabilities of dbt models and apply them to two models: completed_orders
and not_completed_orders
. Through hands-on practice, you’ll gain a solid understanding of how dbt models work. By the end of this tutorial, you will have all the essential knowledge needed to work with dbt models effectively.
To get the most out of this tutorial, make sure to go through the previous one on Install dbt in local. This will ensure a smooth learning experience as you practice along with the exercises.
What’s dbt model? #
First, let’s answer the fundamental question: What is a model in dbt?
A dbt model is a SQL file stored in the models/
directory. It defines transformations applied to raw datasets, typically using a SELECT
query to structure, clean, or aggregate data according to specific business logic.
While Python-based transformations are also possible in dbt, this functionality is only supported by specific database adapters.
Steps to create a model #
Now that we understood what’s dbt model, let’s define the steps required to create a model. The typical steps to create a model in a dbt project are as follows:
- Create a
models/
directory inside your dbt project (if it doesn’t already exist). - Add a new
.sql
file inside themodels/
directory. - Write the SQL query inside the newly created file.
- Configure materialization strategy.
- Run the command
dbt run
to create and execute the model.
Creating a model #
Now, we’ll create a model named completed_orders
by adding a file models/completed_orders.sql
and inserting the SQL statement bellow inside.
The SQL statement bellow retrieve all completed orders from a table demo.orders
SELECT order_id, customer_id, order_date, total_amount FROM demo.orders WHERE status = 'Completed'
💡 When creating a models note that:
- The name of the SQL file will automatically define the name of the table or view in the data source. Therefore, it is important to ensure that the filename is correctly chosen to match the expected structure.
- It is recommended to avoid using dots (
.
) in filenames, as this can cause issues in certain database environments. Instead, underscores (_
) should be used. For example,models/completed_orders.sql
is the correct format, whereasmodels/completed.orders.sql
should be avoided.
Now, run the command bellow to materialze the model.
dbt run
After executing the dbt run
command, dbt materialized the model as view.
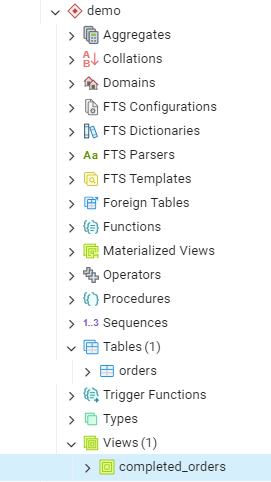
Materialization Strategies #
Materializations are methods for storing dbt models in a data warehouse. dbt includes five built-in materialization types, which are:
Materialization | Definition | Pros | Cons |
---|---|---|---|
view | dbt recreates the model as a view on each run using a CREATE VIEW AS statement. | – No additional storage is required. – Views always reflect the latest records from the source data. | – Slow query performance for complex transformations or when stacking multiple views. |
table | dbt rebuilds the model as a table on each run using a CREATE TABLE AS statement. | – Provide fast query performance. | – Rebuilding tables can be time-consuming, especially for complex transformations. – New records in the source data do not update automatically in the table. |
incremental | Enables dbt to insert or update records in a table only since the last time the model was executed. | – Faster build times by processing only new records instead of the entire dataset. | – Requires additional configuration and is considered an advanced dbt feature. |
ephemeral | Models are not stored in the database. Instead, dbt inlines their code into dependent models using a Common Table Expression (CTE). | – Supports reusable logic while keeping the data warehouse clean by reducing clutter. | – Cannot be queried directly or referenced in dbt run-operation macros. – Overuse can make debugging queries difficult. – Does not support model contracts. |
materialized view | Enables the creation and management of materialized views in the target database. These views combine the benefits of tables and views, making them useful for incremental-style use cases. | – Faster query performance like tables, while maintaining data freshness like views. – Automatically refreshes on a schedule (depending on the database), unlike incremental models that require manual dbt runs. | – Limited configuration options, as support varies by database platform. – Not universally supported across all databases. |
Configure Materialization #
Now, let’s explore how materialization is configured in dbt. By default, models are materialized as views when created. However, you can customize this behavior using three different approaches:
In the configuration file #
Materialization settings can be defined in dbt_project.yml
. The example below specifies that all models within the models/orders
directory will be materialized as views, while those under models/consumers
will be stored as tables:
# In this example config, we tell dbt to build all models in the example/ # directory as views. These settings can be overridden in the individual model # files using the `{{ config(...) }}` macro. models: demo_project: # Config indicated by + and applies to all files under models/example/ orders: +materialized: view consumers: +materialized: table
Model file #
Materialization can also be configured directly in the same .sql
file where the model is defined. In the example below, the model completed_orders is materialized as a table:
{{ config(materialized='table') }} SELECT order_id, customer_id, order_date, total_amount FROM raw.orders WHERE status = 'completed'
Using the properties file #
You can also define materializations in the model’s properties.yml
file. In the example below, the model not_completed_orders is materialized as a view:
version: 2 models: - name: not_completed_orders config: materialized: view
Conclusion #
In this tutorial, you will learn about dbt models, the different strategies for defining them, and the various ways to materialize them in a data warehouse.
Models are the core components of dbt, and mastering them enables you to build scalable, efficient, and maintainable data transformation workflows. Understanding how to configure and materialize models properly will help you optimize query performance, storage, and data freshness in your data pipeline.