In this tutorial, we’ll explore Apache Iceberg, a powerful table format for managing large-scale datasets in data lakes. This is your introduction to Apache Iceberg, where you’ll learn:
- Why Apache Iceberg was designed.
- What Apache Iceberg is.
- How Iceberg addresses common data lake problems, exploring its capabilities, such as schema evolution, hidden partitioning, and time travel.
By the end of this tutorial, you’ll have a clear understanding of what Iceberg is, what it isn’t, and when to use it to optimize your data workflows.
What’s Table Format ? #
A table format is a structured approach to organizing a dataset’s files, allowing them to be represented as a single, cohesive table. From a user’s perspective, it serves as the answer to the question: “What data does this table contain?”
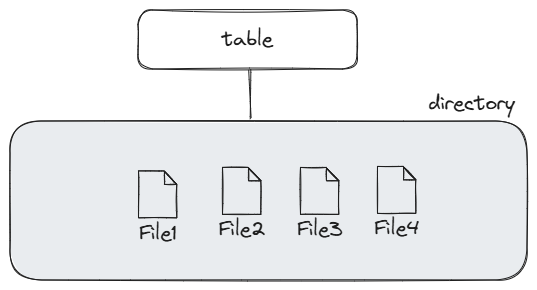
Why Apache Iceberg was designed? #
For a long time, Apache Hive became the de facto table format in data lakes world. It provided several advantages, such as:
- Broad compatibility – Works with almost all data engines due to its long-standing standardization.
- Optimized query performance – Allows more efficient access patterns compared to full-table scans.
- File format flexibility – Supports multiple file formats, making it adaptable to various use cases.
However, despite these benefits, Hive-based table formats had several limitations, including:
- Inefficient for small updates – Making even minor changes required rewriting entire files.
- No safe multi-partition modifications – Changing data across multiple partitions was not reliable.
- Data inconsistency risks – Multiple jobs modifying the same dataset could lead to corruption or conflicts.
- Slow metadata operations – Retrieving directory listings for large tables was time-consuming.
- Complex physical data management – Users needed to understand the underlying table structure to optimize queries.
To overcome these challenges, Apache Iceberg was developed as a next-generation table format. It introduces better schema evolution, hidden partitioning, snapshot-based operations, and transactional consistency, solving many of the inefficiencies of traditional Hive tables. It does also bring several other features and optimizations.
What is Apache Iceberg? #
Now as you understood why Iceberg was developed, let’s address the question what’s Apache Iceberg. It is:
- A table format specification that defines how data is stored, structured, and managed within a table.
- A set of APIs and libraries designed to facilitate interaction with the table format.
- These libraries integrate with various data processing engines and tools, enabling them to read, write, and manage Iceberg tables efficiently.
- Compatible with widely used big data processing engines such as Apache Spark, Trino, Flink, and Hive.
Note that Iceberg Is Not (❌):
- A Service: It is not a separate running service or database but a format specification that integrates with other data platforms.
- A Storage Engine: It does not handle physical data storage directly. Instead, it works with existing storage solutions like Amazon S3, HDFS, or Google Cloud Storage.
- An Execution Engine: Iceberg does not perform query execution or data processing. It relies on engines like Apache Spark, Trino, Presto, and Flink to process data.
Iceberg Benefits #
- Schema Evolution: Allows for seamless schema evolution, overcoming the challenges associated with changes in data structure over time.
- Transactional Writes: By supporting transactional writes, Iceberg ensures the atomicity, consistency, isolation, and durability (ACID) properties, enhancing data integrity during write operations.
- Query Isolation: Iceberg provides query isolation, preventing interference between concurrent read and write operations, thus improving overall system reliability and performance.
- Time Travel: The time travel feature in Iceberg allows users to access historical versions of the data, offering a valuable mechanism for auditing, analysis, and debugging.
- Partition Pruning: Iceberg’s partition pruning capability optimizes query performance by selectively scanning only relevant partitions, reducing the amount of data processed and improving query speed.
Conclusion #
In this introductory tutorial to Apache Iceberg, you’ve learned what Iceberg is and explored its key features that make it a powerful solution for managing large-scale data in modern data lakes.
To deepen your understanding and get hands-on experience, check out our practical guide: 👉 Getting Started with Apache Iceberg.